Image, Video and 3D Content AIGC Models
Our research focuses on advancing Image, Video, and 3D Content Generation through cutting-edge AI-Generated Content (AIGC) models, with a primary emphasis on diffusion models. These models have demonstrated remarkable potential in generating high-quality, realistic visual and 3D content. By leveraging the power of diffusion processes, we aim to create systems capable of synthesizing intricate, contextually coherent content across multiple modalities.
Our ultimate goal is to contribute to the development of a world model—a comprehensive, generative understanding of the environment and its dynamics. This involves not only producing visually stunning and realistic content but also ensuring that the generated outputs align with complex real-world structures and behaviors. Through our research, we seek to push the boundaries of content creation technologies, enabling new applications in entertainment, virtual reality, and digital interaction. By bridging generative capabilities with the broader vision of a world model, we aim to unlock unprecedented possibilities for creativity and innovation.
Equilibrated Diffusion: Frequency-aware Textual Embedding for Equilibrated Image Customization
Equilibrated Diffusion draws inspiration from the correlation between high- and low-frequency components with image style and content, decomposing concept accordingly in the frequency domain. Through independently optimizing concept embeddings in the frequency domain, the denoising model not only enriches its comprehension of style attribute irrelevant to subject identity but also inherently augments its aptitude for accommodating novel stylized descriptions.
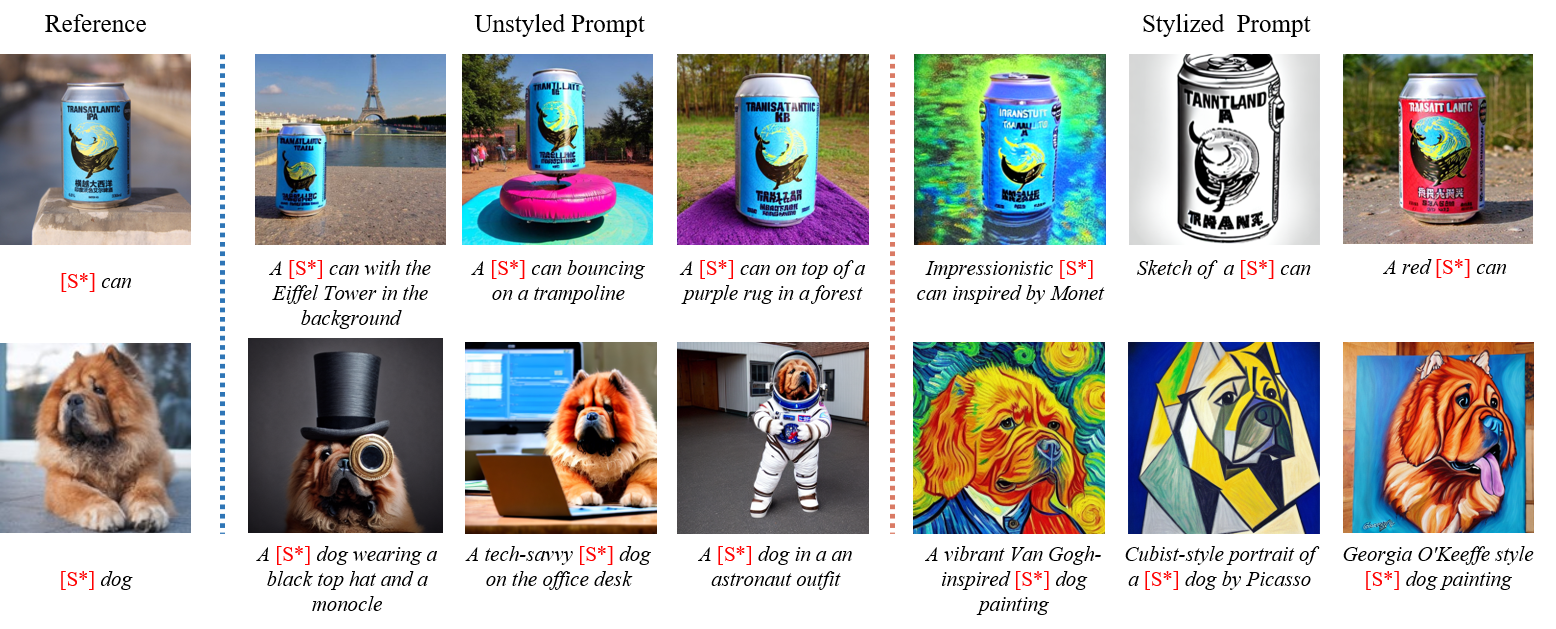
Video Generation for World Modeling
Video generation aims to produce realistic and dynamic visual content, with the ultimate goal of simulating real-world dynamics and interactions. This capability allows us to build a virtual simulator of the real world. Such a simulator holds great potential as it can provide valuable priors for numerous tasks that require knowledge about the physical world. By leveraging this technology, researchers can address challenges in fields such as robotics, autonomous systems, and other domains where understanding and predicting real-world behaviors are crucial.
OmniMotionGPT: Animal Motion Generation with Limited Data
Our paper aims to generate diverse and realistic animal motion sequences from textual descriptions, without a large-scale animal text-motion dataset. While the task of text-driven human motion synthesis is already extensively studied and benchmarked, it remains challenging to transfer this success to other skeleton structures with limited data. In this work, we design a model architecture that imitates Generative Pretraining Transformer (GPT), utilizing prior knowledge learned from human data to the animal domain. We jointly train motion autoencoders for both animal and human motions and at the same time optimize through the similarity scores among human motion encoding, animal motion encoding, and text CLIP embedding. Presenting the first solution to this problem, we are able to generate animal motions with high diversity and fidelity, quantitatively and qualitatively outperforming the results of training human motion generation baselines on animal data.
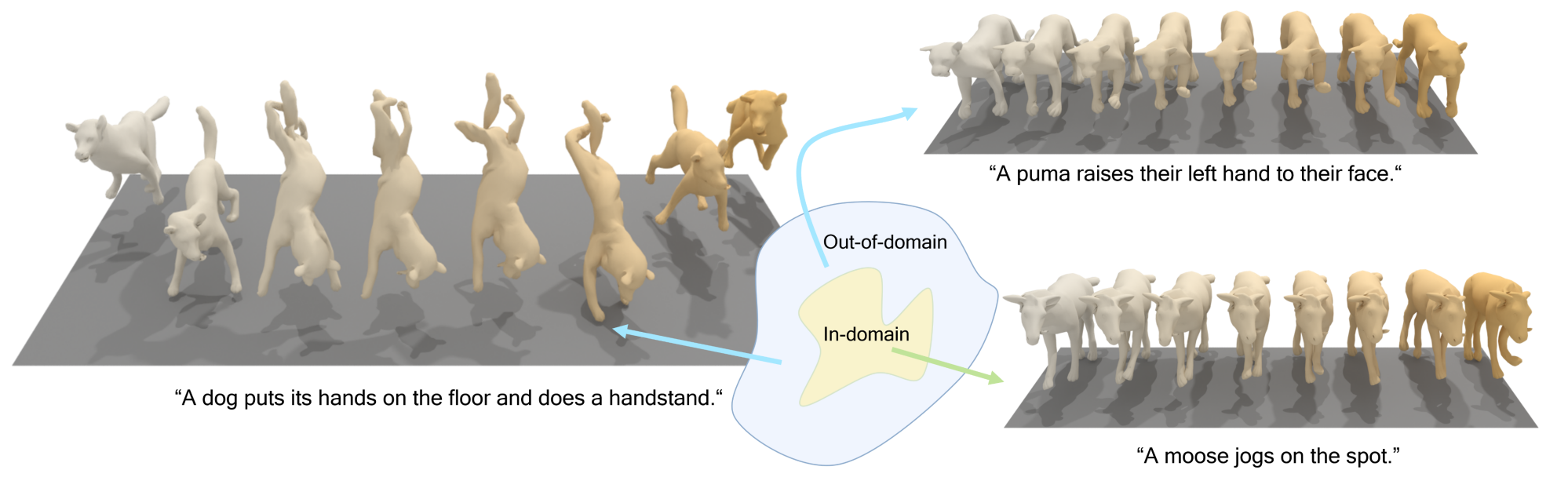